- Understanding Linear Programming
- Real-Life Applications
- Setting Up Your LP Problem in MS Excel
- Practical Example: Reducing Transportation Costs
- Advanced LP Techniques in Excel
- Tips and Tricks for LP in Excel
- Case Study: Cutting Manufacturing Costs
- Conclusion
In the realm of business and operations management, cost reduction is a primary objective for many organizations. To achieve this, companies often turn to mathematical optimization techniques like Linear Programming (LP). LP is a powerful tool that allows organizations to make informed decisions by minimizing costs or maximizing profits, subject to certain constraints. In this blog, we'll explore the world of LP models and how students can leverage MS Excel to solve assignments related to cost reduction using LP techniques. Cost reduction is a critical aspect of modern business operations. Whether it's a manufacturing company seeking to cut production costs, a logistics company optimizing transportation expenses, or a financial institution managing investments more efficiently, the ability to reduce costs can significantly impact an organization's profitability and competitiveness. This is where Linear Programming (LP) enters the scene as a valuable problem-solving tool. LP is a mathematical approach to decision-making that helps organizations allocate resources optimally to achieve specific goals, such as cost reduction. If you need assistance with your economics assignment, understanding LP models and their practical applications can provide valuable insights into optimizing business processes and driving financial efficiency.
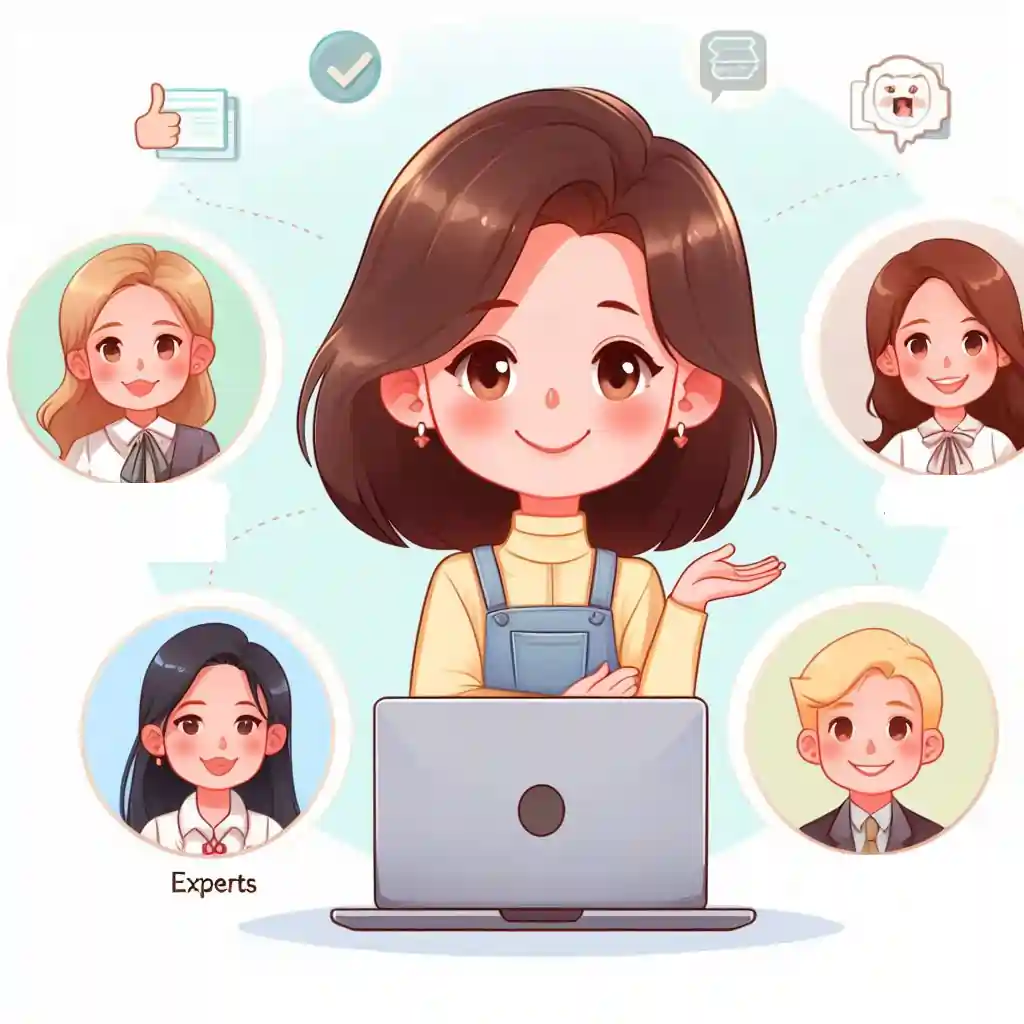
LP models consist of three main components: decision variables, an objective function, and constraints. Decision variables represent the choices or levels an organization wants to determine, such as production quantities, investment amounts, or allocation of resources. The objective function defines the goal of the optimization, whether it's minimizing costs, maximizing profits, or achieving some other objective. Constraints are limitations or restrictions on the decision variables, reflecting real-world limitations like budget constraints, resource availability, or demand requirements. When all these components are integrated, LP models provide a structured way to find the best possible solution, balancing competing objectives while adhering to practical constraints.
Students studying business, engineering, economics, or related fields can greatly benefit from understanding and applying LP techniques to real-world problems. Moreover, they can harness the power of Microsoft Excel, a widely accessible and user-friendly spreadsheet software, to solve LP assignments and gain practical experience. Excel offers a built-in Solver tool that allows users to formulate and solve LP problems effortlessly. This combination of LP knowledge and Excel skills equips students with a valuable set of tools for addressing complex decision-making challenges they might encounter in their future careers.
Throughout this blog, we will delve into various aspects of LP and its application in cost reduction scenarios. We will explore real-life applications where LP can be a game-changer, such as supply chain optimization, portfolio management, and inventory cost reduction. We'll also provide a step-by-step guide on how to set up and solve LP problems using Microsoft Excel, making it accessible and actionable for students. To illustrate these concepts in a practical context, we will present case studies and examples of cost reduction problems, demonstrating how LP models can be constructed and solved in Excel.
Understanding Linear Programming
- What is Linear Programming?
- Linear Programming Components
- Decision Variables: These represent the quantities or levels you want to find. For cost reduction, these might be production levels, allocation of resources, or purchasing decisions.
- Objective Function: This defines what you're trying to minimize or maximize, typically a cost or profit function.
- Constraints: These are limitations on decision variables, like budget constraints, production capacity, or demand requirements.
- Cost Reduction Scenarios
- Transportation Cost Reduction: Optimizing routes and vehicle allocation for transportation companies.
- Manufacturing Cost Reduction: Optimizing production plans to minimize costs.
- Inventory Cost Reduction: Determining optimal inventory levels to reduce holding costs.
- Supply Chain Optimization
- Supplier selection and allocation.
- Inventory management.
- Transportation logistics.
- Portfolio Optimization
- Preparing Data
- Formulating the LP Problem
- Solving LP Problems in MS Excel
- Problem Statement
- Data Collection and Preparation
- Formulating the LP Model
- Solving in MS Excel
- Sensitivity Analysis
- Integer Linear Programming (ILP)
- Goal Programming
- Data Validation and Error Checking
- Solver Options and Settings
- Problem Description
- Data Gathering and Preparation
- Formulating the LP Model
- Solving in MS Excel
Understanding Linear Programming is crucial in solving complex optimization problems. Linear Programming (LP) is a mathematical technique used to find the best possible outcome in a given mathematical model, primarily characterized by linear relationships between variables. At its core, LP involves defining decision variables, constructing an objective function to either maximize profits or minimize costs, and subjecting these variables to constraints. The objective is to find values for the decision variables that optimize the objective function while satisfying all constraints. This methodology finds broad applications in various fields, including finance, manufacturing, logistics, and even everyday decision-making. For students, grasping the fundamentals of LP equips them with a powerful problem-solving tool. It enables them to make informed decisions, allocate resources efficiently, and tackle real-world challenges by translating them into mathematical models. Ultimately, understanding Linear Programming empowers individuals and organizations to achieve better outcomes, whether it's cost reduction, profit maximization, or resource allocation.
Linear Programming is a mathematical technique used to find the best outcome in a mathematical model with linear relationships. It helps in optimizing resource allocation, whether it's minimizing costs, maximizing profits, or achieving a combination of objectives. LP models consist of an objective function and a set of constraints.
To understand LP, you must grasp its components:
Real-Life Applications
Real-life applications of linear programming models are ubiquitous across various industries, playing a pivotal role in optimizing operations and reducing costs. In logistics and transportation, these models enable companies to streamline routes, allocate resources efficiently, and minimize fuel expenses, ultimately enhancing overall cost-effectiveness. The manufacturing sector benefits from linear programming by optimizing production schedules, resource allocation, and inventory management, leading to reduced production costs. Moreover, in finance and investment, linear programming aids in constructing diversified portfolios that balance risk and return, a crucial aspect of cost-effective investment strategies. Supply chain management leverages these models to make informed decisions regarding supplier selection, inventory control, and demand forecasting, resulting in reduced supply chain costs. From healthcare resource allocation to energy production and even in agriculture for crop management, linear programming proves to be an invaluable tool for decision-makers, helping them make cost-efficient choices and allocate resources optimally in the complex landscape of real-world problems.
Linear programming finds applications in various industries to reduce costs. Examples include:
Supply chain management involves multiple decision points where cost reduction can be achieved. LP can optimize:
In finance, LP can be used to optimize investment portfolios, balancing risk and return to reduce costs while achieving financial objectives.
Setting Up Your LP Problem in MS Excel
Setting up your Linear Programming (LP) problem in MS Excel involves several key steps to transform real-world challenges into mathematical models. To embark on this journey, begin by meticulously collecting and preparing your data, ensuring that it accurately reflects the problem's nuances. Organize your information into well-structured tables or lists, adding clear labels for clarity. Next, you'll need to formulate the LP model. This entails defining your decision variables, constructing an objective function that either minimizes costs or maximizes profits, and establishing constraints that mirror real-world limitations, such as budget constraints, production capacity, or demand requirements. MS Excel's formula bar becomes your canvas for this mathematical translation. Lastly, harness the power of Excel's built-in Solver tool, configuring it to find the optimal solution for your LP model. With Solver's assistance, Excel transforms your data and formulas into actionable insights, allowing you to make informed decisions and, ultimately, achieve cost reduction or profit maximization objectives.
When embarking on data preparation, the initial step involves gathering and systematically structuring your data within the Excel environment. Begin by establishing tables or lists that accommodate decision variables, coefficients, and constraints. It is imperative to incorporate clear labeling and meticulous formatting techniques to enhance data comprehensibility, ultimately facilitating a streamlined process for subsequent analysis and modeling.
Translate your real-world problem into mathematical equations. Define your decision variables, create an objective function, and set up constraints using Excel's formula bar.
Excel has a built-in tool called Solver that can solve LP problems. Configure Solver by specifying the objective function to minimize or maximize, decision variables, and constraints. Excel will find the optimal solution.
Practical Example: Reducing Transportation Costs
Imagine you work for a logistics company facing the common challenge of minimizing transportation costs while efficiently meeting customer demand. This practical scenario necessitates the application of Linear Programming (LP) techniques in conjunction with MS Excel to derive cost-effective solutions. To illustrate, gather data on the locations of warehouses, delivery points, associated shipping costs, and customer demand. With this information, construct an LP model in Excel. In this model, you'll define decision variables representing the quantities to be shipped from each warehouse to specific delivery locations. The objective function will aim to minimize transportation expenses, while constraints will be set based on warehouse capacities and delivery demands. Executing Solver in Excel will then yield the optimal allocation strategy, showcasing how LP in Excel can significantly reduce transportation costs, enhance operational efficiency, and deliver real-world benefits to logistics companies striving for cost-effective solutions.
Imagine you work for a delivery company, and you want to minimize transportation costs while meeting customer demand. You have multiple warehouses and delivery locations, each with different shipping costs and demand.
Gather data on warehouse locations, delivery locations, shipping costs, and demand. Organize this data into Excel.
Define decision variables, create an objective function to minimize transportation costs, and set constraints based on warehouse capacity and delivery demand.
Use Solver to find the optimal solution. Excel will provide you with the optimal allocation of goods from warehouses to delivery locations, minimizing transportation costs.
Advanced LP Techniques in Excel
In the realm of operations research and decision science, mastering advanced Linear Programming (LP) techniques in Microsoft Excel elevates one's ability to tackle complex optimization challenges. Beyond the basic LP applications, delving into advanced methods opens doors to even more refined solutions. Sensitivity analysis, a crucial technique, allows practitioners to gauge the robustness of their solutions by exploring how changes in coefficients and constraints impact the outcome, providing valuable insights for decision-makers. Integer Linear Programming (ILP) extends the scope of LP by permitting variables to assume only integer values, making it indispensable for problems where decisions must be whole numbers, such as quantities of items in inventory or the number of production shifts. Moreover, Goal Programming caters to situations where cost reduction is not the sole objective; it enables the simultaneous pursuit of multiple goals, striking a delicate balance between various objectives. With these advanced LP techniques at your disposal, Excel becomes an indispensable tool for precise and informed decision-making in diverse real-world scenarios.
Perform sensitivity analysis to understand how changes in coefficients and constraints impact your LP solution.
ILP extends LP by allowing decision variables to take only integer values. It's useful for problems where decisions must be whole numbers, such as quantities of items.
Goal Programming handles multiple objectives simultaneously. It can be used when cost reduction is not the sole objective, but you also want to achieve other goals.
Tips and Tricks for LP in Excel
When venturing into the realm of Linear Programming (LP) within Microsoft Excel, it's crucial to arm yourself with some valuable tips and tricks that can significantly enhance your efficiency and effectiveness. First and foremost, meticulous data validation and error checking should be an ongoing practice, ensuring that your input data is accurate and consistent, as even minor discrepancies can lead to erroneous results. Secondly, don't underestimate the power of Solver's options and settings; experimenting with different solving methods and precision settings can yield more accurate and faster solutions to your LP problems. Additionally, when tackling complex LP models, consider breaking them down into smaller, more manageable sub-problems, solving them individually, and then integrating the solutions to arrive at an overall optimal solution. Finally, always perform sensitivity analysis to understand the robustness of your solution concerning changes in coefficients and constraints, allowing you to make more informed decisions and adapt to dynamic environments effectively.
Ensure data accuracy and consistency by using Excel's data validation and error-checking features.
Experiment with different Solver options and settings to fine-tune your LP solutions. Try different solving methods and precision settings.
Case Study: Cutting Manufacturing Costs
In our comprehensive case study titled "Cutting Manufacturing Costs," we delve into the strategic efforts of a manufacturing company determined to enhance its cost-efficiency while simultaneously fulfilling customer demand across a diverse product range. This scenario presents a complex challenge that often confronts businesses in today's competitive landscape. Our study begins with a meticulous data collection process, where we gather essential information about production costs, demand patterns, resource availability, and capacity constraints. Subsequently, we employ Microsoft Excel as a powerful tool to construct a Linear Programming model. This model integrates decision variables for production levels, an objective function aimed at minimizing production costs, and a set of constraints grounded in the company's resource capabilities and market requirements. By harnessing the computational prowess of MS Excel, we illustrate how businesses can systematically optimize their production plans, effectively curtailing expenditures while meeting consumer demand, and ultimately positioning themselves for greater competitiveness and sustainability in their respective industries.
Consider a manufacturing company aiming to reduce production costs while meeting customer demand for multiple products.
Collect data on production costs, demand, resource availability, and capacity constraints. Organize this data in Excel.
Define decision variables for production levels, create an objective function to minimize production costs, and set constraints based on resource availability and demand.
Utilize Solver to find the optimal production plan, minimizing costs while satisfying demand and resource constraints.
Conclusion
In the case study focused on cutting manufacturing costs, we delve into the challenges faced by a manufacturing company striving to enhance its cost-efficiency while simultaneously meeting the diverse demands of its product portfolio. The study entails meticulous data gathering and organization, encompassing crucial variables such as production costs, demand fluctuations, resource availability, and capacity constraints. To address this multifaceted problem, the study employs the powerful methodology of Linear Programming within the familiar framework of MS Excel. Decision variables are meticulously defined to represent production levels, with an objective function aimed at minimizing production costs without compromising product demand fulfillment. Stringent constraints, grounded in resource constraints and customer demand, are set to reflect real-world limitations. Through the sophisticated solving capabilities of MS Excel's Solver tool, the case study illustrates how the manufacturing company can strategically optimize its production plan, ultimately achieving the twin goals of cost reduction and customer satisfaction in a highly competitive market landscape. This case study serves as an exemplary model of how mathematical optimization techniques can be practically harnessed to attain tangible cost-saving outcomes in real-world manufacturing scenarios.