- Understanding the Foundation: Econometrics and Forecasting
- Econometrics Essentials
- The Significance of Forecasting in Econometrics
- Data Preparation and Cleaning
- Quality over Quantity
- Time Series Data
- Model Selection and Specification
- Choosing the Right Model
- Model Specification
- Estimation Techniques
- Parameter Estimation
- Diagnostic Testing
- Validation and Evaluation
- Out-of-Sample Testing
- Performance Metrics
- Interpretation and Communication
- Interpreting Results
- Effective Communication
- Continuous Learning and Improvement
- Stay Updated with Method
- Seek Feedback
- Conclusion
Mastering the art of forecasting in econometrics requires a seamless integration of economics, mathematics, and statistics, providing a powerful tool for understanding and dissecting economic phenomena. In the realm of university assignments, where students often grapple with complex datasets and intricate models, the significance of forecasting cannot be overstated. It stands as a linchpin skill, guiding students through the labyrinth of econometric analyses. This comprehensive guide aims to unravel the intricacies of forecasting, offering a treasure trove of tips and insights designed to bolster students' confidence and proficiency in handling their assignments. As we embark on this journey, it is crucial to recognize the foundational importance of econometrics itself—a discipline that amalgamates theoretical economic concepts with the precision of mathematical and statistical tools. At the heart of econometrics lies forecasting, a predictive prowess that enables us to anticipate trends, plan strategically, and make informed decisions based on empirical evidence. As students delve into this multifaceted field, understanding the nuances of forecasting becomes paramount, akin to mastering the strokes of a complex painting. The guide will navigate through various stages, beginning with the essential step of data preparation and cleaning. Here, the mantra is quality over quantity, emphasizing the importance of accurate, error-free datasets that form the bedrock of reliable forecasting. Time series data, often encountered in econometric assignments, adds another layer of complexity, demanding an appreciation of temporal dimensions, seasonality, and cyclical patterns. If you find yourself in need of assistance with your econometrics homework, this guide is tailored to offer practical help and support throughout your learning process.
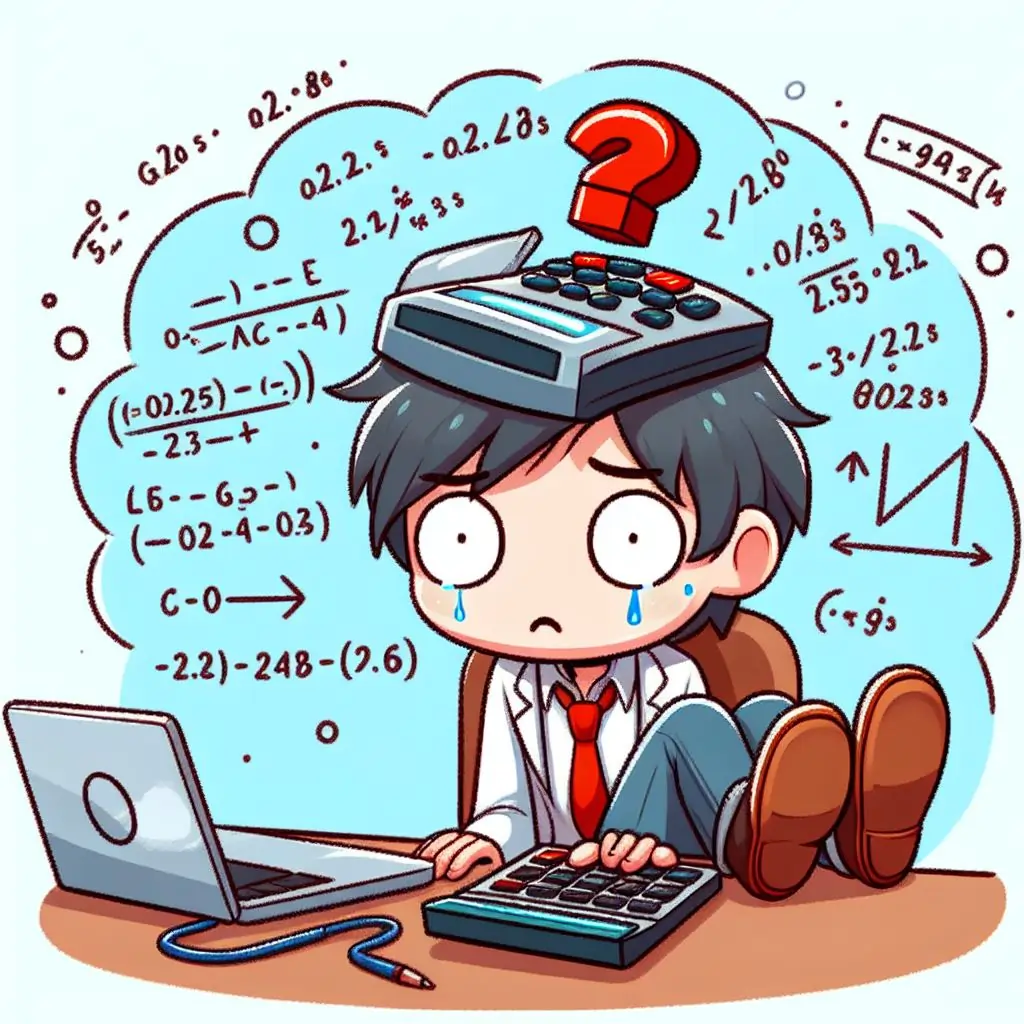
Moving forward, the guide addresses the critical juncture of model selection and specification. Choosing the right model and meticulously defining its variables lay the groundwork for accurate forecasting. Parameter estimation techniques, such as Ordinary Least Squares, come into play, along with diagnostic testing to ensure the model's validity. The validation and evaluation phase introduces the necessity of out-of-sample testing, safeguarding against overfitting and validating the model's predictive prowess. Performance metrics like Mean Squared Error and Mean Absolute Error become the litmus test for forecasting accuracy. Beyond the technicalities, effective interpretation and communication of results are emphasized, encouraging students to articulate findings coherently using visual aids for enhanced clarity. The guide culminates in a call for continuous learning and improvement, underscoring the dynamic nature of econometrics and the need to stay abreast of evolving methods. Seeking feedback from mentors and peers becomes a cornerstone for refining forecasting skills, transforming students into adept practitioners of the art of forecasting in the intricate landscape of econometrics. As the guide concludes, the aim is for students to not only conquer their assignments but to emerge as adept forecasters, equipped to navigate the real-world complexities of economics and decision-making.
Understanding the Foundation: Econometrics and Forecasting
Understanding the foundation of econometrics and forecasting is paramount for any student venturing into the intricate world of economic analysis. Econometrics, at its core, serves as the intersection of economics, mathematics, and statistics, providing a robust framework for comprehending and analyzing economic phenomena. In this realm, forecasting emerges as a pivotal skill, indispensable for navigating the challenges posed by university assignments. As students delve into the first segment of this comprehensive guide, they must grasp the essential elements that form the backbone of econometrics. This involves acquiring a nuanced understanding of regression analysis, hypothesis testing, and model specifications, as these concepts lay the groundwork for accurate and effective forecasting. The significance of forecasting in econometrics cannot be overstated, as it not only aids in predicting future trends but also facilitates strategic decision-making based on empirical evidence. Therefore, before embarking on the intricacies of forecasting models and techniques, students must solidify their understanding of econometrics as the discipline that equips them with the tools to bridge theoretical economic concepts with the precision of mathematical and statistical methods. As they progress through this foundational stage, students will be well-prepared to tackle subsequent aspects of the guide, armed with the knowledge necessary to navigate the complexities of data preparation, model selection, parameter estimation, and validation processes inherent in the art of forecasting within the discipline of econometrics.
Econometrics Essentials
Embarking on the journey of forecasting within econometrics necessitates a solid foundation in its essentials. Before students can delve into the intricacies of predicting future trends, they must first master the fundamental principles that underpin econometric analyses. These include a comprehensive understanding of regression analysis, a statistical technique that explores relationships between variables, hypothesis testing, which allows for the validation or rejection of proposed hypotheses based on data, and model specifications, where the structure and components of a model are defined. Together, these elements lay the groundwork for constructing models that facilitate accurate and effective forecasting, making them indispensable building blocks for any student navigating the complex terrain of econometrics.
The Significance of Forecasting in Econometrics
The significance of forecasting in the realm of econometrics extends far beyond mere statistical predictions. It emerges as a dynamic tool with the power to shape decisions for businesses, governments, and individuals. Forecasting acts as a compass, guiding strategic planning and enabling proactive responses to anticipated trends. Businesses can optimize production and marketing strategies, governments can formulate effective policies, and individuals can make informed choices about investments or personal finances. As a result, the ability to forecast becomes a linchpin skill, bridging the gap between historical data and future outcomes, empowering decision-makers to navigate uncertainties with confidence. Understanding the pivotal role of forecasting sets the stage for students to appreciate its real-world implications, ensuring that as they progress in their econometric endeavors, they recognize the broader impact their analytical skills can have on diverse sectors of the economy.
Data Preparation and Cleaning
In the intricate landscape of econometrics, the significance of meticulous data preparation and cleaning cannot be overstated. This second segment of our guide underscores the pivotal role that quality data plays in the forecasting process. As students venture into the realm of econometric assignments, the mantra becomes clear: quality over quantity. The journey begins with the recognition that the accuracy and reliability of forecasts hinge on the integrity of the data used for analysis. This involves a rigorous process of cleaning the dataset, where outliers are identified and addressed, missing values are carefully managed, and variables are transformed if necessary. The goal is to cultivate a dataset that is not only accurate but also devoid of errors that could skew results. Moreover, when dealing with time series data, a common occurrence in econometrics, an additional layer of complexity is introduced. Students are encouraged to delve into the temporal dimension, considering seasonality, trends, and cyclical patterns that may influence the data. Visual exploration through graphs and charts emerges as a valuable tool in gaining insights into the characteristics of the data. As students navigate through this stage of the guide, they are equipped with the skills to discern between noise and meaningful patterns within the data. Ultimately, understanding the intricacies of data preparation and cleaning becomes the cornerstone for robust econometric analyses, laying a solid foundation for the subsequent stages of model selection, estimation, and validation in the pursuit of mastering the art of forecasting.
Quality over Quantity
In the realm of econometrics, the axiom "quality over quantity" takes center stage as students embark on the crucial phase of data preparation. The foundation for accurate and insightful forecasting lies in the integrity of the dataset. Therefore, it is imperative to commence with high-quality data, ensuring its accuracy, relevance, and freedom from errors. Cleaning the data is not a mere formality but a fundamental step, demanding meticulous attention. This process includes the identification and removal of outliers that could distort results, addressing missing values to prevent bias, and, when necessary, transforming variables to enhance the dataset's suitability for analysis. This emphasis on data quality sets the stage for a robust and reliable forecasting model, positioning students for success in the intricate world of econometrics.
Time Series Data
As students delve deeper into econometric assignments, a common encounter is with time series data, a dynamic form that adds an additional layer of complexity to the forecasting process. Understanding the temporal dimension is paramount, urging students to consider inherent factors such as seasonality, trends, and cyclical patterns. Visualization becomes a powerful ally in this endeavor, as exploring time series data through graphs and charts provides valuable insights into its characteristics. By immersing themselves in the visual representation of data, students can discern patterns and anomalies, laying the groundwork for more informed and nuanced analyses. This section serves as a crucial bridge, guiding students from the general principles of data preparation to the specific considerations required when dealing with the temporal intricacies of econometric datasets.
Model Selection and Specification
Entering the domain of econometrics, the journey advances to the pivotal stage of model selection and specification. Here, the trajectory toward mastering the art of forecasting solidifies as students navigate the nuanced process of choosing the right model and meticulously defining its components. Model selection is a strategic decision-making process, akin to selecting the appropriate tool for a specific task. It requires a profound understanding of the dataset and the dynamics of the economic phenomena under examination. Various models, such as ARIMA, VAR, or regression models, stand at the disposal of the econometrician, each with its strengths and limitations. Students must adeptly align the model with the nature of their data, recognizing the unique characteristics that demand a tailored approach. Moving beyond selection, model specification becomes the next frontier. This involves precisely defining the variables that influence the dependent variable and determining the lag structures, particularly crucial in time series data. A well-specified model is not only theoretically sound but also instrumental in producing meaningful forecasting results. As students navigate this segment of the guide, they are urged to exercise a judicious blend of theoretical knowledge and practical acumen, ensuring that their chosen model aligns harmoniously with the intricacies of the data. This phase acts as a crucial crossroads, marking the transition from foundational principles to the intricate nuances of econometric modeling. As students master the art of model selection and specification, they pave the way for the subsequent stages of estimation, validation, and interpretation, propelling them toward proficiency in the dynamic realm of forecasting within the realm of econometrics.
Choosing the Right Model
In the intricate landscape of econometrics, the crux of forecasting lies in the judicious selection of an appropriate model. This critical decision-making process demands a nuanced understanding of various models, including but not limited to ARIMA, VAR, and regression models. Each model carries its unique strengths and limitations, and the key is to align the choice with the inherent characteristics of the dataset. Recognizing the dynamics of the economic phenomena under scrutiny, students must navigate through the complexities, ensuring that the chosen model harmonizes seamlessly with the nature of their data. The selection process is more than a technical exercise; it is a strategic decision that lays the foundation for accurate and insightful forecasting.
Model Specification
Having embarked on the path of model selection, the journey in econometrics converges on the critical juncture of model specification. Here, precision and careful consideration take precedence. Defining the model involves a meticulous process wherein variables influencing the dependent variable are explicitly specified, and for time series data, lag structures are considered. The essence lies in the details; a well-specified model is not just theoretically robust but is a linchpin for generating meaningful and reliable forecasting results. This phase demands a careful balance between theoretical knowledge and practical application, urging students to delve into the intricacies of their chosen model. As they navigate through the landscape of model specification, students lay the groundwork for a forecasting framework that transcends theoretical soundness to practical utility, marking a significant stride towards mastering the art of forecasting in the realm of econometrics.
Estimation Techniques
As the econometric journey progresses, the spotlight turns to the crucial realm of estimation techniques, a domain where theoretical understanding converges with practical application. At the heart of accurate forecasting lies the process of parameter estimation, a methodical endeavor essential for unveiling the relationships embedded within the chosen model. Ordinary Least Squares (OLS), a widely employed technique, becomes a cornerstone in this phase, as it facilitates the estimation of model parameters by minimizing the sum of squared differences between observed and predicted values. As students delve into the nuances of estimation, it becomes paramount to comprehend the assumptions underpinning these techniques, ensuring that the results drawn from the estimation process are not only statistically significant but also robust. Diagnostic testing emerges as an integral companion in this journey, allowing students to assess the validity of their model. Heteroscedasticity, autocorrelation, and multicollinearity are scrutinized to identify potential pitfalls that might compromise the reliability of forecasting outcomes. Beyond mere estimation, this phase underscores the importance of a comprehensive understanding of the chosen model's dynamics, enabling students to interpret coefficients, assess the direction and magnitude of effects, and recognize any underlying assumptions. As the econometrician navigates through this stage, a holistic grasp of estimation techniques becomes a powerful tool, not only for producing accurate forecasts but also for gaining profound insights into the intricate relationships governing economic phenomena. With each estimation, students inch closer to mastering the art of forecasting within the dynamic tapestry of econometrics, equipped with the skills to unravel the complexities of data and transform theoretical constructs into tangible insights for decision-makers.
Parameter Estimation
Having meticulously specified the chosen model, the econometrician transitions into the realm of parameter estimation, a critical phase that bridges theoretical constructs with empirical reality. Once armed with a well-defined model, the estimation process unfolds, aiming to unravel the relationships embedded within. Ordinary Least Squares (OLS), a prevalent method, takes center stage, facilitating the estimation of parameters by minimizing the sum of squared differences between observed and predicted values in linear regression models. However, the efficacy of parameter estimation hinges on a profound understanding of the assumptions inherent in these techniques. Students are urged to delve into the intricacies, ensuring not only technical proficiency but also the ability to make valid inferences that withstand the scrutiny of empirical testing.
Diagnostic Testing
With parameters estimated and the model seemingly in place, the journey through econometrics encounters a pivotal checkpoint—diagnostic testing. This phase is instrumental in scrutinizing the validity of the model, safeguarding against potential pitfalls that could compromise the reliability of forecasting results. Diagnostic tests serve as a vigilant gatekeeper, scrutinizing issues such as heteroscedasticity, autocorrelation, and multicollinearity. Identifying and rectifying these concerns is not just an exercise in refinement; it is a crucial step in ensuring that the model faithfully captures the underlying economic relationships. As students perform diagnostic tests, they fortify the robustness of their forecasts, recognizing that the reliability of predictions rests on the foundation of a thoroughly validated model. In essence, diagnostic testing becomes the guardian of econometric integrity, assuring that the forecasting journey is not just technically proficient but also anchored in the empirical soundness of the chosen model.
Validation and Evaluation
As the econometric voyage continues, the focus shifts to the critical phases of validation and evaluation—cornerstones for ensuring the credibility and robustness of forecasting endeavors. Validation becomes an imperative checkpoint, demanding that the forecasted model stands up to the scrutiny of real-world scenarios. Out-of-sample testing emerges as a potent tool, utilizing data not employed in the initial estimation process to assess the model's predictive accuracy. This strategic validation process guards against overfitting and ensures the generalizability of the model beyond the confines of the training data. Simultaneously, performance metrics, such as Mean Squared Error (MSE), Mean Absolute Error (MAE), or Root Mean Squared Error (RMSE), take center stage in the evaluation arena. These metrics provide quantitative insights into the forecasting accuracy, serving as benchmarks to gauge the effectiveness of the model. As students traverse through the realms of validation and evaluation, they gain not only a profound understanding of the model's predictive power but also an appreciation for the nuanced interplay between theory and empirical outcomes. The thorough examination of forecasting performance underscores that the true mastery of econometrics extends beyond theoretical constructs to the tangible impact of predictions in the real world. It is a synthesis of empirical validation, metric evaluation, and an unwavering commitment to producing forecasts that transcend mere academic exercises, becoming valuable tools for strategic decision-making in the dynamic landscapes of economics and business. In essence, the validation and evaluation phases epitomize the culmination of the art of forecasting in econometrics, where theoretical prowess converges with real-world applicability, defining a pathway for students to navigate the complexities of their assignments with acumen and confidence.
Out-of-Sample Testing
The journey through econometrics reaches a pivotal juncture with the introduction of out-of-sample testing, a strategic validation process essential for gauging the robustness and generalizability of forecasting models. As students transition from the intricacies of model specification and parameter estimation, the focus now extends beyond the training dataset. Out-of-sample testing involves validating the model using data not utilized during the initial estimation process. This deliberate separation of data aims to scrutinize the model's predictive accuracy and its ability to transcend the confines of the training set. By subjecting the model to scenarios unseen during estimation, students gain insights into its resilience and adaptability, safeguarding against the risk of overfitting and ensuring a more reliable application in real-world situations.
Performance Metrics
The evaluation of forecasting performance takes center stage in the econometric landscape, with students delving into the intricacies of performance metrics to quantify the accuracy of their predictions. Metrics such as Mean Squared Error (MSE), Mean Absolute Error (MAE), and Root Mean Squared Error (RMSE) emerge as the yardsticks for assessing forecasting efficacy. Understanding these metrics transcends mere numerical calculations; it equips students with the language to communicate the precision of their forecasts effectively. Each metric offers unique insights into different aspects of forecasting performance, enabling a comprehensive evaluation. Mastery of these performance metrics is not just a technical exercise but a key to unlocking the potential of econometric forecasts, providing students with the tools to convey the real-world implications of their analyses accurately. As students navigate through the intricacies of out-of-sample testing and performance metrics, they emerge with a holistic understanding of forecasting—where theoretical constructs meet empirical validation, setting the stage for proficient decision-making in the dynamic landscapes of economics and data analysis.
Interpretation and Communication
With forecasting outcomes in hand, the econometrician enters the crucial phase of interpretation and communication, where the art of turning numbers into actionable insights comes to the forefront. The results gleaned from the forecasting model are not mere statistical outcomes but windows into the intricacies of economic phenomena. Interpretation becomes an intellectual journey, demanding a discerning eye to unravel the meaning behind coefficients, understand the direction and magnitude of effects, and discern any underlying assumptions. This phase is where theory meets the tangible, as the econometrician draws connections between the model's outputs and real-world implications. Communication emerges as the bridge that connects the intricate web of econometric analysis with decision-makers, stakeholders, or a broader audience. Visual aids, such as graphs and charts, become invaluable tools in articulating the complex relationships unearthed by the model. Effectively conveying the implications of forecasting results is not only about technical prowess but also about storytelling—the ability to distill complex data into a narrative that resonates with the intended audience. As students navigate through the realms of interpretation and communication, they not only refine their analytical skills but also cultivate the art of translating data-driven insights into compelling narratives. This transformative process marks the convergence of the technical and the practical, where the proficiency to not only interpret but also effectively communicate forecasting outcomes becomes the hallmark of a skilled econometrician. In essence, interpretation and communication transcend the confines of academic exercises, empowering students to bridge the gap between complex analyses and informed decision-making in the dynamic landscapes of economics and business.
Interpreting Results
As the curtain rises on the results of a forecasting model, the econometrician assumes the role of an interpreter, decoding the intricate language of statistical outputs into meaningful insights. The clarity of interpretation becomes paramount at this juncture, demanding a comprehensive understanding of the significance of coefficients. Through a discerning lens, the econometrician assesses the direction and magnitude of the effects unveiled by the model, navigating the intricacies of economic relationships. Simultaneously, a keen awareness is required to identify any limitations or assumptions inherent in the forecasting process. This interpretative phase is not merely a technical exercise but an intellectual journey where theory meets the tangible outcomes, allowing for a nuanced understanding of the economic phenomena under scrutiny.
Effective Communication
The art of effective communication emerges as the linchpin for translating forecasting insights into actionable knowledge. Beyond mastering the intricacies of the model, the econometrician steps into the role of a storyteller, articulating findings in a coherent narrative. Visual aids, such as graphs and charts, become potent tools in this communicative arsenal, enhancing the clarity of the presentation. The challenge lies not only in technical precision but in the ability to convey the implications of forecasts to decision-makers in a manner that resonates. Effectiveness in communication is the conduit through which the intricate web of econometric analysis reaches a broader audience. Through compelling narratives and visually appealing representations, the econometrician bridges the gap between the complexities of data and the pragmatic needs of those tasked with making informed decisions in the dynamic landscapes of economics and business. In essence, effective communication transforms statistical outcomes into a powerful tool for strategic decision-making, elevating the impact of econometric analyses beyond the confines of academia.
Continuous Learning and Improvement
As the journey through the realms of econometrics unfolds, the final act reveals itself in the imperative of continuous learning and improvement—an ethos that distinguishes adept practitioners from novices. In this ever-evolving field, marked by dynamic economic landscapes and emerging methodologies, the commitment to ongoing education becomes a cornerstone. Beyond mastering the techniques outlined in earlier stages, students are encouraged to stay updated with the latest advancements and methodologies in econometrics. This involves a relentless pursuit of knowledge, embracing new theories, models, and tools that shape the discipline. Seeking feedback becomes not just a means of validation but a catalyst for improvement, inviting constructive insights from professors, peers, or industry professionals. The econometrician's toolkit expands beyond static models and formulae to a dynamic arsenal informed by the cutting edge of research. Embracing continuous learning is not merely a professional obligation but a pathway to mastery in the art of forecasting. The iterative process of refining skills, adapting to evolving landscapes, and incorporating feedback positions the econometrician as a perpetual learner, resilient in the face of uncertainty and change. This ethos extends beyond academic endeavors, preparing students for the demands of real-world applications where adaptability and a commitment to staying at the forefront of econometric advancements are paramount. In essence, the concluding chapter of continuous learning and improvement serves as an invitation—a perpetual call for exploration, growth, and the unwavering pursuit of excellence in the dynamic and ever-unfolding narrative of econometrics.
Stay Updated with Method
In the ever-evolving realm of econometrics, staying updated with methods is not just a best practice; it is an imperative for adept practitioners. The field is dynamic, marked by continual advancements and the introduction of novel techniques. Students are urged to remain at the forefront of these developments, cultivating a mindset of perpetual learning. As new methods emerge, whether in model specifications, estimation techniques, or diagnostic tools, staying informed becomes the key to maintaining the relevance and effectiveness of forecasting endeavors. This commitment to staying abreast of the latest methodologies ensures that the econometrician's toolkit is not stagnant but evolves with the discipline itself.
Seek Feedback
In the pursuit of mastering the art of forecasting in econometrics, seeking feedback is a linchpin for growth and improvement. This involves actively reaching out to professors, peers, and industry professionals to solicit constructive criticism. Feedback is more than a validation; it is a catalyst for refining skills and enhancing the accuracy of forecasts. Through external perspectives, students gain valuable insights into areas for improvement, potential blind spots, and nuances that may have been overlooked. Constructive feedback not only sharpens technical proficiency but also fosters a mindset of continuous improvement. In the dynamic landscape of econometrics, where the real-world implications of forecasts matter, the ability to incorporate feedback becomes a distinguishing factor, elevating the precision and applicability of the econometrician's analyses. Seeking feedback is not just a step in the learning process; it is an ongoing commitment to excellence and a recognition that growth is a collaborative and iterative journey.
Conclusion
Mastering the art of forecasting in econometrics is a journey that demands a solid understanding of the foundational concepts, meticulous data preparation, thoughtful model selection, and continuous learning. By following these tips, students can navigate their university assignments with confidence, producing forecasts that are not only academically sound but also valuable for real-world decision-making. Embrace the challenges, learn from experiences, and let the art of forecasting in econometrics become a skill that sets you apart in the world of economics and data analysis.
You Might Also Like
Delve into a trove of knowledge and insights with our captivating blogs. From expert tips to industry trends and innovative solutions, discover fresh perspectives on topics that resonate with you. Stay informed, inspired, and empowered as you explore our latest posts.