- Understanding Quantile Regression:
- Why Quantile Regression Matters
- Robustness to Outliers:
- Capturing Distributional Changes:
- Handling Non-Normal Residuals:
- Applications of Quantile Regression:
- Income Inequality:
- Labor Market Studies:
- Health Economics:
- Finance:
- How to Implement Quantile Regression:
- Choose the Quantile(s):
- Estimation Methods:
- Interpretation of Results:
- Diagnostic Tests:
- Conclusion
Econometrics, situated at the crossroads of economics and statistics, assumes a pivotal role in unraveling and modeling the intricate relationships within the economic realm. As students embark on their journey into the complex realm of econometrics, they grapple with an array of statistical tools designed to facilitate the analysis and interpretation of economic data. Amidst this toolkit, Quantile Regression emerges as a particularly influential instrument, garnering increasing recognition in recent years. The primary objective of this blog post is to furnish students with a comprehensive comprehension of Quantile Regression, shedding light on its versatile applications and demonstrating its efficacy in tackling intricate challenges embedded within university assignments. As the demand for sophisticated statistical methodologies intensifies, grasping the nuances of Quantile Regression not only equips students with a powerful analytical tool but also opens up new avenues for insightful contributions to the dynamic field of econometrics. This post seeks to bridge the gap between theoretical knowledge and practical application, empowering students to harness the potential of Quantile Regression for a more nuanced understanding of economic phenomena and the proficient resolution of complex problems in the academic sphere. Through exploration of its robustness to outliers, ability to capture distributional changes, and adept handling of non-normal residuals, students will gain insights into the diverse advantages that Quantile Regression brings to the forefront. Delving into real-world applications, such as income inequality analysis, labor market studies, investigations in health economics, and financial modeling, this post aims to illustrate the versatility of Quantile Regression across various domains. If you need assistance with your econometrics homework, don't hesitate to reach out. I'm here to provide support and guidance to ensure your success.
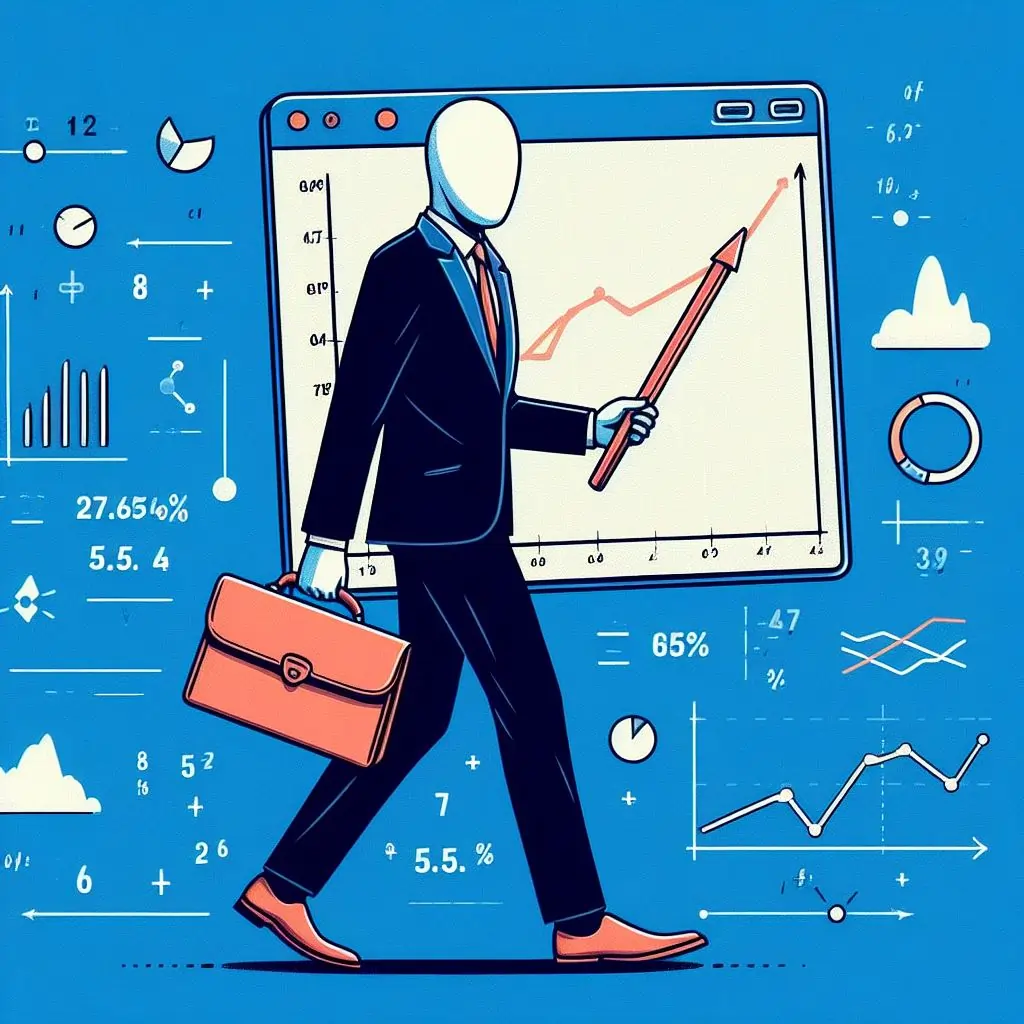
Additionally, the implementation process is demystified, guiding students through the crucial steps of choosing quantiles, selecting estimation methods, interpreting results, and performing diagnostic tests to ensure the model's validity. A practical example involving the relationship between education and income serves as a tangible illustration of how Quantile Regression transcends traditional approaches by offering a more nuanced understanding of heterogeneous effects across different income levels. As students navigate their university assignments, armed with a newfound appreciation for Quantile Regression, they are poised to elevate the depth and precision of their analyses. In conclusion, as Quantile Regression solidifies its status as an indispensable tool in the econometrician's repertoire, this blog post endeavors to empower students with the knowledge and skills necessary to leverage its potential effectively, fostering a generation of economists and researchers capable of navigating the evolving landscape of empirical research with confidence and insight.
Understanding Quantile Regression:
Quantile Regression stands as a formidable statistical technique, expanding the horizons of traditional linear regression by facilitating the exploration of diverse quantiles of the dependent variable. In contrast to conventional regression models that predominantly concentrate on predicting the mean or average value of the dependent variable, quantile regression introduces a nuanced approach. It empowers researchers to dissect the conditional distribution of the response variable at various points, offering a granular perspective on how different segments of the data behave. This inherent flexibility positions quantile regression as an invaluable tool for capturing the heterogeneity present in datasets, a facet often obscured by the more simplistic assumptions of traditional regression models. By allowing for a more detailed examination of the distribution, quantile regression equips analysts with the means to discern and comprehend variability across different quantiles, providing a richer and more accurate representation of the underlying relationships within the data. This adaptability is particularly crucial in instances where the effects of independent variables exhibit variations across distinct segments of the population or dataset. As a result, quantile regression emerges as an indispensable analytical tool, opening new avenues for researchers and students alike to delve into the complexities of data and derive meaningful insights that may be overlooked by more conventional modeling approaches.
Why Quantile Regression Matters
Quantile Regression holds significant importance for various reasons within the realm of econometrics. Firstly, its robustness to outliers distinguishes it from traditional regression models, which are often sensitive to extreme values. By focusing on specific points in the distribution, Quantile Regression provides a more resilient and accurate analysis, mitigating the impact of outliers on the estimated coefficients. Secondly, the capability of capturing distributional changes is crucial for understanding how different segments of the dependent variable respond to changes in independent variables. This versatility allows researchers to delve into nuanced patterns and uncover heterogeneity that may go unnoticed in mean-focused analyses. Additionally, the flexibility of Quantile Regression in handling non-normal residuals enhances its applicability to a broader range of real-world datasets, where the normality assumption may not hold. As econometricians and researchers grapple with increasingly complex data, the unique strengths of Quantile Regression make it an indispensable tool for uncovering hidden insights and addressing the multifaceted challenges inherent in empirical studies.
Robustness to Outliers:
Quantile Regression offers a crucial advantage in its robustness to outliers, a feature that sets it apart from traditional linear regression models. Extreme values have the potential to exert a disproportionate impact on estimated coefficients in conventional models, leading to skewed and unreliable results. In contrast, quantile regression focuses on specific points in the distribution, making it less susceptible to the influence of outliers. This inherent robustness enhances the accuracy of the model, providing econometricians with a more reliable representation of the underlying data.
Capturing Distributional Changes:
The unique strength of Quantile Regression lies in its ability to capture distributional changes by examining different quantiles of the dependent variable. Each quantile represents a distinct point in the distribution, allowing economists to discern how various parts of the dependent variable's distribution respond to alterations in independent variables. This approach provides a nuanced and comprehensive understanding of the data, particularly beneficial in situations where the effects of certain variables exhibit variations across different segments of the population. The flexibility to analyze multiple quantiles enriches the analysis, uncovering hidden patterns that may remain obscured in mean-focused models.
Handling Non-Normal Residuals:
In the realm of traditional linear regression, the assumption of normally distributed residuals is often made. However, real-world data frequently deviates from this idealized norm. Quantile Regression steps in as a more flexible alternative, adept at handling non-normal residuals. This adaptability renders it suitable for a broader spectrum of empirical studies, where the assumption of normality may not align with the actual characteristics of the data. As researchers grapple with the complexities of diverse datasets, Quantile Regression stands as a valuable tool for accommodating the intricacies of real-world residual distributions, ensuring the robustness and reliability of the analytical process.
Applications of Quantile Regression:
Quantile Regression, with its unique ability to unveil nuanced relationships within data, finds applications across diverse domains, enhancing the depth and precision of empirical studies. One prominent application lies in the analysis of income inequality, where researchers employ quantile regression to investigate how economic policies or external factors impact various income groups differently. By examining different quantiles of the income distribution, a more detailed understanding of disparities emerges, allowing for targeted policy recommendations. In the realm of labor market studies, quantile regression proves instrumental in dissecting wage differentials. Analysts can discern whether factors such as education or experience have varying effects on different segments of the wage distribution, providing insights crucial for informed labor market policies. Health economics benefits from the versatility of quantile regression by studying the impact of socioeconomic factors on health outcomes at different points in the health distribution. This approach acknowledges the heterogeneous nature of health outcomes and guides the formulation of tailored interventions. Furthermore, in finance, quantile regression serves as a valuable tool for modeling and forecasting financial returns. Investors can assess risk and potential rewards across various quantiles of return distributions, aiding in the development of robust investment strategies. The broad applicability of quantile regression is evident as it transcends disciplinary boundaries, offering a flexible and powerful approach to analyzing complex relationships within diverse datasets. As students engage with university assignments, understanding these practical applications equips them with the tools to apply quantile regression in real-world scenarios, fostering a deeper appreciation for its relevance and utility in empirical research.
Income Inequality:
Quantile Regression emerges as a powerful tool in the analysis of income inequality, offering a nuanced perspective on the differential impacts of economic policies or external factors on various income groups. Through the examination of distinct quantiles within the income distribution, researchers can discern subtle variations in the effects of these factors across different strata of society. This approach facilitates a more granular understanding of income disparities, enabling policymakers to formulate targeted interventions that address specific segments of the population. The application of Quantile Regression in income inequality studies thus contributes to more informed and equitable economic policy decisions.
Labor Market Studies:
Within the realm of labor economics, Quantile Regression proves indispensable in unraveling the complexities of wage differentials across the income distribution. By dissecting how factors such as education or experience influence different segments of the wage structure, researchers can provide insights that transcend traditional mean-focused analyses. This nuanced understanding of wage differentials informs labor market policies, guiding the development of strategies that address the diverse needs of workers across various income levels. Quantile Regression, in this context, becomes a key analytical tool for crafting targeted and effective labor market interventions.
Health Economics:
In health economics, Quantile Regression plays a pivotal role in studying the impact of socioeconomic factors on health outcomes at various points in the health distribution. This approach acknowledges the inherent variability in health outcomes and provides a more comprehensive understanding of the complex relationships between variables. Researchers can identify how specific factors affect different segments of the population, allowing for the development of more tailored and effective health policies. The versatility of Quantile Regression in health economics contributes to a more holistic and nuanced approach to addressing public health challenges.
Finance:
The realm of finance leverages Quantile Regression for modeling and forecasting financial returns, adding a layer of sophistication to risk assessment and investment strategy development. By analyzing different quantiles of return distributions, investors gain insights into the varying levels of risk and potential rewards associated with different investment strategies. This application of Quantile Regression equips financial analysts and investors with a more nuanced understanding of the potential outcomes and uncertainties in the financial markets. The ability to account for different quantiles enhances the robustness of financial models, allowing for more informed decision-making in an ever-changing economic landscape.
How to Implement Quantile Regression:
Implementing Quantile Regression involves a systematic process that empowers researchers to extract valuable insights from data at various points in the distribution. The first crucial step is to decide which quantile or quantiles of the dependent variable to analyze. This decision depends on the research question at hand and the characteristics of the data. Once the quantiles are selected, the next step is to choose an estimation method. Various methods, including iterative procedures and optimization algorithms, are available for estimating quantile regression models. Popular statistical software packages such as R, Python (utilizing libraries like statsmodels or scikit-learn), and Stata provide tools and functions for implementing these estimation methods. Interpretation of the results is another vital aspect of implementing Quantile Regression. Unlike traditional regression models where coefficients represent average effects, in Quantile Regression, coefficients signify the changes in the specific quantile of interest. Understanding how these coefficients vary across quantiles is crucial for drawing meaningful conclusions about the relationship between independent and dependent variables at different points in the distribution. Additionally, conducting diagnostic tests is imperative to ensure the validity of the model. Residual analysis, tests for heteroscedasticity, and goodness-of-fit tests aid in assessing whether the assumptions of the quantile regression model hold. The implementation process, from selecting quantiles to interpreting results and conducting diagnostic tests, demands a blend of statistical knowledge and software proficiency. Through mastering the practical aspects of Quantile Regression, researchers and students can unlock the full potential of this powerful technique, contributing to more accurate and nuanced analyses in econometric studies and empirical research.
Choose the Quantile(s):
The initial step in the implementation of Quantile Regression requires a thoughtful decision on which quantile or quantiles of the dependent variable to analyze. This choice is pivotal and hinges upon the specifics of the research question at hand and the distributional characteristics inherent in the data under scrutiny. Determining the relevant quantiles sets the stage for a nuanced exploration of how the relationships between variables vary across different segments of the data distribution, providing a more detailed and context-specific understanding.
Estimation Methods:
After identifying the quantiles of interest, the subsequent stage involves selecting appropriate estimation methods. Numerous methodologies exist for estimating quantile regression models, encompassing iterative procedures and optimization algorithms. Well-established statistical software packages such as R, Python (leveraging libraries like statsmodels or scikit-learn), and Stata offer efficient tools for executing these estimation methods. Familiarity with these tools empowers researchers to navigate the intricate landscape of quantile regression modeling, extracting meaningful insights from their data.
Interpretation of Results:
Interpreting the outcomes of quantile regression constitutes a critical aspect of the implementation process. Unlike traditional regression models where coefficients signify average effects, understanding the variations in coefficients across different quantiles is paramount. This nuanced interpretation unveils how the relationship between independent and dependent variables evolves at distinct points in the distribution, providing researchers with valuable insights into the heterogeneity of these relationships.
Diagnostic Tests:
Ensuring the robustness and validity of the quantile regression model demands the execution of diagnostic tests. Residual analysis, tests for heteroscedasticity, and goodness-of-fit tests serve as essential tools for evaluating the performance of the model and verifying the adherence to underlying assumptions. Rigorous diagnostic testing enhances the reliability of the insights derived from quantile regression analyses, instilling confidence in the validity and accuracy of the results. As researchers navigate the intricacies of diagnostic testing, they fortify the foundation of their quantile regression models, ensuring a robust and trustworthy analytical framework.
Conclusion
Quantile Regression, with its ability to capture distributional changes and provide a robust analysis of heterogeneous effects, stands as a powerful tool in the econometrician's toolkit. As students navigate through their econometrics assignments, incorporating quantile regression can enhance the depth and accuracy of their analyses. By understanding the applications, implementation, and interpretation of quantile regression, students can unlock new avenues in empirical research and contribute to the evolving field of econometrics. As the demand for nuanced and sophisticated statistical techniques grows, mastering Quantile Regression becomes a valuable skill for aspiring economists and researchers alike.
Similar Blogs
Enhance your understanding of economics with our expert guidance at economicshomeworkhelper.com. Our blog offers insightful articles, practical tips, and detailed explanations to help you excel in your studies. Stay ahead with the latest economic trends and ace your assignments with ease!