Avail Your Discount Now
Discover an amazing deal at www.economicshomeworkhelper.com! Enjoy a generous 10% discount on all economics homework, providing top-quality assistance at an unbeatable price. Our team of experts is here to support you, making your academic journey more manageable and cost-effective. Don't miss this chance to improve your skills while saving money on your studies. Grab this opportunity now and secure exceptional help for your economics homework.
We Accept
- Understanding the Basics
- Assumptions of Multivariate Regression:
- Data Preparation and Variable Selection
- Estimation and Interpretation
- Assessing Model Fit and Significance
- Dealing with Multicollinearity
- Dealing with Multicollinearity
- Heteroscedasticity and Residual Analysis
- Model Validation and Prediction
- Real-world Applications and Case Studies
- Conclusion
Econometrics, the intersection of economics, statistics, and mathematics, plays a pivotal role in understanding and interpreting economic phenomena, serving as a bridge between theoretical economic models and empirical data. Among the many tools in the econometrician's arsenal, multivariate regression analysis stands out as a powerful and widely used method for exploring relationships between multiple variables, allowing researchers to investigate complex economic dynamics and make informed policy recommendations. In this handbook, we will delve into the intricacies of multivariate regression analysis, providing students with a comprehensive guide to tackle their econometrics homework with confidence. By mastering the principles and techniques of multivariate regression, students can unlock a deeper understanding of economic relationships and phenomena, equipping them with the analytical skills necessary to address real-world economic challenges. Throughout this guide, we will explore the underlying assumptions of multivariate regression, the steps involved in model specification and estimation, techniques for assessing model fit and significance, and strategies for dealing with common issues such as multicollinearity and heteroscedasticity. Moreover, we will emphasize the importance of data preparation and variable selection, highlighting the need for careful consideration and theoretical grounding in the selection of independent variables. Drawing on examples from economics literature and empirical research, we will demonstrate how multivariate regression analysis can be applied to a wide range of economic questions, from studying the determinants of economic growth to analyzing the impact of policy interventions on consumer behavior.
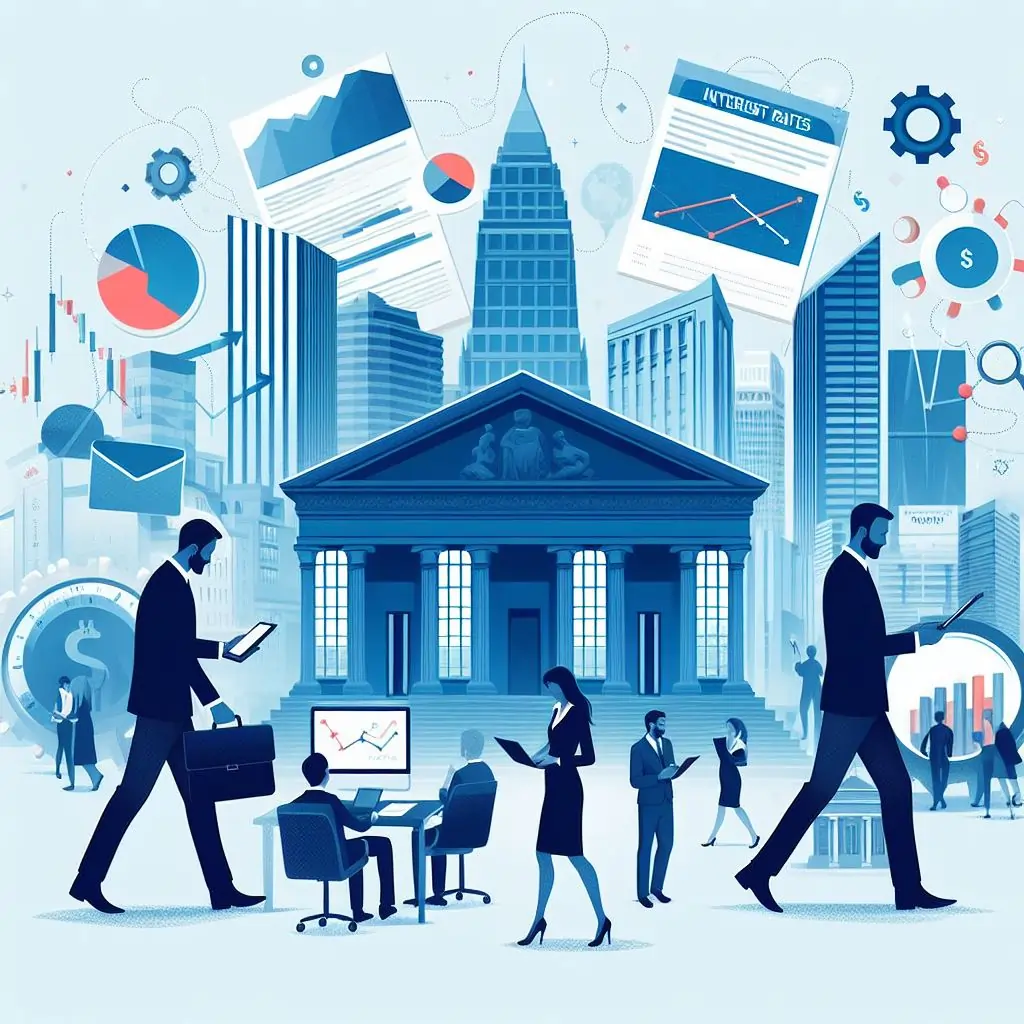
By providing clear explanations, step-by-step instructions, and practical tips, this handbook aims to empower students to confidently apply multivariate regression analysis to their own research projects and homework. Whether you are a novice econometrics student or an experienced researcher looking to deepen your understanding of multivariate regression, this handbook offers valuable insights and resources to support your learning journey. From interpreting regression coefficients to diagnosing model misspecification, each chapter is designed to build upon the foundational concepts of econometrics, guiding you through the process of conducting rigorous and meaningful empirical analysis. Ultimately, mastering multivariate regression analysis is not just about acquiring technical skills; it is about developing a critical mindset and a deep appreciation for the complexities of economic data and analysis. By engaging with the material presented in this handbook and applying it to your own research projects, you will gain the confidence and competence needed to contribute to the ongoing dialogue in the field of econometrics and economics more broadly.
Understanding the Basics
Understanding the basics of regression analysis is crucial for grasping the complexities of multivariate regression. Simple linear regression serves as the cornerstone, focusing on the relationship between two variables: the dependent variable (Y) and the independent variable (X). This straightforward model provides a starting point for understanding how changes in one variable relate to changes in another. However, real-world economic phenomena are rarely so simplistic; they are typically influenced by a multitude of factors. Multivariate regression expands upon the framework of simple linear regression by allowing us to consider the impact of multiple independent variables on a dependent variable simultaneously. By incorporating additional explanatory variables into the analysis, multivariate regression enables a more nuanced understanding of the underlying relationships driving economic outcomes. This broader perspective is essential for capturing the multifaceted nature of economic phenomena and making more accurate predictions and policy recommendations. Thus, before embarking on multivariate regression analysis, it is essential to have a solid understanding of simple linear regression and its fundamental principles. Building upon this foundation, students can then explore the intricacies of multivariate regression, unlocking new insights into the complex interplay between variables in economic systems.
The Multivariate Regression Model
A multivariate regression model is expressed as follows:
Yi=β0+β1X1i+β2X2i+...+βkXki+ϵi
Here,
Yi is the dependent variable for observation i,
β0 is the intercept term,
β1,β2,...,βk are the coefficients associated with the independent variables X1i,X2i,...,Xki,
ϵi is the error term.
Assumptions of Multivariate Regression:
- Linearity: The relationship between the dependent and independent variables is linear. This assumption implies that changes in the independent variables have a constant effect on the dependent variable.
- Independence: The residuals (ϵ i) are independent of each other. This assumption ensures that there is no systematic pattern or correlation among the residuals, indicating that each observation provides unique information.
- Homoscedasticity: The variance of residuals is constant across all levels of the independent variables. Homoscedasticity indicates that the spread of residuals remains consistent across the range of predictor variables, allowing for reliable estimation of coefficients.
- No perfect multicollinearity: The independent variables are not perfectly correlated. Perfect multicollinearity occurs when one independent variable can be perfectly predicted from the others, leading to unreliable coefficient estimates.
- Normality of residuals: Residuals should be normally distributed. This assumption suggests that the errors or residuals follow a normal distribution, which facilitates the application of inferential statistical tests and ensures the validity of parameter estimates.
Data Preparation and Variable Selection
Data preparation and variable selection are foundational steps in conducting a successful multivariate regression analysis. Meticulous data preparation is paramount, as clean and organized data serve as the bedrock for deriving meaningful insights. This process entails handling missing values, detecting and addressing outliers, and transforming variables if necessary to meet the assumptions of the regression model. Additionally, variable selection plays a crucial role in the analysis. It is imperative to consider the economic theory underpinning the relationships being investigated to identify relevant independent variables. However, striking the right balance is essential; including too many variables can lead to overfitting, where the model captures noise rather than true relationships, while including too few variables may result in an oversimplified model that fails to capture the complexity of the underlying phenomenon. Thus, careful consideration and theoretical grounding are necessary when selecting independent variables to ensure that the chosen model effectively captures the dynamics of the relationship under study without introducing unnecessary complexity or bias. By adhering to best practices in data preparation and variable selection, researchers can lay a solid foundation for conducting rigorous multivariate regression analyses and deriving robust conclusions from their empirical investigations.
Estimation and Interpretation
Estimation and interpretation are fundamental stages in multivariate regression analysis, following meticulous data preparation. Once the dataset is cleaned and organized, the focus shifts to estimation, wherein various methods, including the widely used Ordinary Least Squares (OLS) method, are employed to estimate the coefficients of the regression model. These coefficients represent the magnitude and direction of the relationship between the independent variables and the dependent variable. Interpreting these coefficients is crucial for understanding the impact of each independent variable on the dependent variable. A positive coefficient suggests a positive relationship, indicating that an increase in the independent variable leads to an increase in the dependent variable, while a negative coefficient implies an inverse relationship. The magnitude of the coefficient reflects the strength of the relationship, with larger coefficients indicating a more substantial impact. Additionally, interpreting coefficients allows researchers to assess the statistical significance of each independent variable, typically through hypothesis testing, such as t-tests or F-tests. Statistical significance indicates whether the observed relationship between the independent and dependent variables is unlikely to have occurred by chance alone. Moreover, interpreting coefficients in the context of economic theory and real-world implications enhances the insights derived from the regression analysis, enabling researchers to make informed decisions and policy recommendations based on their findings. Thus, estimation and interpretation are integral components of multivariate regression analysis, enabling researchers to uncover meaningful relationships and draw valid conclusions from their empirical investigations.
Assessing Model Fit and Significance
Assessing the fit and significance of a multivariate regression model is essential to ensure its validity and reliability in explaining the relationships between variables. One commonly used metric for evaluating model fit is the coefficient of determination (R^2), which measures the proportion of variance in the dependent variable that is explained by the independent variables included in the model. A higher R^2 value indicates a better fit, suggesting that the independent variables collectively account for a larger portion of the variability observed in the dependent variable. However, it's important to consider the context of the specific research question and the inherent complexities of the data when interpreting R^2. Additionally, hypothesis tests, such as the F-test, provide valuable insights into the overall significance of the model. The F-test evaluates whether the independent variables, taken together, have a statistically significant effect on the dependent variable. A significant F-test result indicates that the model as a whole provides a better explanation of the variation in the dependent variable compared to a model with no independent variables. By conducting thorough assessments of model fit and significance, researchers can determine the validity and usefulness of their regression models in explaining the underlying relationships between variables. This, in turn, enables them to draw meaningful conclusions and make informed decisions based on the empirical evidence derived from their analyses.
Dealing with Multicollinearity
Multicollinearity, a common issue in regression analysis, occurs when independent variables are highly correlated, which can lead to challenges in interpreting regression results accurately. When multicollinearity is present, it becomes difficult to isolate the individual effects of each independent variable on the dependent variable, as their effects become confounded. This can undermine the reliability of coefficient estimates and inflate standard errors, making it challenging to draw meaningful conclusions from the analysis. To mitigate the impact of multicollinearity, various techniques are employed, with variance inflation factor (VIF) analysis being one of the most commonly used approaches. VIF analysis assesses the extent to which the variance of an estimated regression coefficient is inflated due to multicollinearity, with higher VIF values indicating greater multicollinearity. By identifying independent variables with high VIF values, researchers can pinpoint the variables that are contributing most to multicollinearity and consider potential remedies, such as excluding highly correlated variables from the analysis or combining them into composite variables. Addressing multicollinearity through VIF analysis helps ensure the robustness of the regression model, enhancing the reliability and validity of the results. By effectively managing multicollinearity, researchers can more accurately estimate the effects of independent variables on the dependent variable, enabling them to derive more precise and interpretable insights from their regression analyses.
Dealing with Multicollinearity
Multicollinearity, a common challenge in regression analysis, occurs when independent variables are highly correlated, leading to difficulties in accurately interpreting regression results. When multicollinearity is present, it complicates the estimation of the regression coefficients and inflates their standard errors, making it challenging to discern the individual effects of each independent variable on the dependent variable. This can undermine the reliability and validity of the regression model, hindering the ability to draw meaningful conclusions from the analysis. To address multicollinearity, various techniques are employed, with variance inflation factor (VIF) analysis being one of the most widely used methods. VIF analysis assesses the extent to which the variance of an estimated regression coefficient is inflated due to multicollinearity, with higher VIF values indicating greater multicollinearity. By identifying independent variables with high VIF values, researchers can pinpoint the variables that are contributing most to multicollinearity and consider potential remedies, such as excluding highly correlated variables from the analysis or combining them into composite variables. By effectively managing multicollinearity through techniques like VIF analysis, researchers can enhance the robustness of the regression model and improve the accuracy and interpretability of the results. This enables them to derive more reliable insights from their regression analyses, facilitating informed decision-making and advancing understanding in their respective fields.
Heteroscedasticity and Residual Analysis
Heteroscedasticity, a common issue in regression analysis, refers to the violation of the assumption of constant variance across the range of independent variables, which can have significant implications for the reliability and accuracy of regression results. When heteroscedasticity is present, the standard errors of the regression coefficients may be biased, leading to incorrect inference about the significance of the relationships between variables. To identify and address heteroscedasticity, researchers often employ residual analysis, a critical component of regression diagnostics. Residuals, which are the differences between the observed and predicted values of the dependent variable, are examined using scatterplots and residual plots to detect any patterns or trends that indicate heteroscedasticity. In a scatterplot of residuals against the predicted values, heteroscedasticity is evident if the spread or dispersion of the residuals varies systematically across different levels of the predicted values. Similarly, in a residual plot, heteroscedasticity is indicated by non-random patterns or trends in the distribution of residuals. Once heteroscedasticity is detected, researchers can explore potential remedies, such as transforming the dependent variable or using robust standard errors to adjust for heteroscedasticity. By effectively addressing heteroscedasticity through residual analysis, researchers can improve the reliability and validity of their regression models, ensuring that the estimated relationships between variables are accurately captured and interpreted. This enhances the trustworthiness of the findings and enables more informed decision-making based on the results of the regression analysis.
Model Validation and Prediction
Model validation and prediction are crucial steps in econometrics homework, ensuring the reliability and predictive capability of the regression model. Before finalizing the analysis, it is essential to validate the model to assess its performance in making predictions on new data. One common approach to model validation is to split the dataset into training and testing sets. The training set is used to estimate the parameters of the regression model, while the testing set is used to evaluate the model's predictive performance on unseen data. Various metrics, such as Mean Squared Error (MSE) and Root Mean Squared Error (RMSE), are commonly employed to assess the accuracy of the model's predictions. MSE measures the average squared difference between the actual and predicted values, providing a measure of the model's overall predictive error. Similarly, RMSE provides the square root of the MSE, offering a more interpretable measure of prediction error in the same units as the dependent variable. By comparing the model's predictions against the actual outcomes in the testing set, researchers can evaluate its accuracy and identify any potential shortcomings or areas for improvement. This process of model validation ensures that the regression model is robust and reliable, enhancing confidence in its ability to make accurate predictions on new data. Ultimately, by rigorously validating the model and assessing its predictive performance using appropriate metrics, researchers can derive meaningful insights from their econometrics homework and make informed decisions based on the results of the analysis.
Real-world Applications and Case Studies
Real-world applications and case studies serve as invaluable resources for deepening your understanding of multivariate regression analysis. By examining how economists and researchers have applied this technique in practice to address complex problems and extract meaningful insights from data, you can gain a richer appreciation for its utility and versatility. Real-world applications encompass a wide range of domains, from finance and marketing to healthcare and environmental science, demonstrating the broad applicability of multivariate regression analysis across diverse fields. Case studies offer concrete examples of how multivariate regression has been used to analyze complex datasets, identify patterns and relationships, and inform decision-making. Through these case studies, you can observe the practical challenges encountered in real-world applications, such as data collection issues, model specification dilemmas, and interpretation nuances, and learn how researchers have navigated and overcome these challenges. Moreover, real-world applications and case studies provide opportunities to explore the limitations of multivariate regression analysis and the importance of contextual factors in interpreting regression results. By critically engaging with real-world examples, you can develop a deeper understanding of the strengths and limitations of multivariate regression analysis and hone your ability to apply it effectively in your own research or professional endeavors. Additionally, by synthesizing insights from multiple case studies across different domains, you can gain a more comprehensive understanding of the broader impact and relevance of multivariate regression analysis in addressing real-world problems and advancing knowledge in various fields. Therefore, to solidify your understanding of multivariate regression analysis, immerse yourself in real-world applications and case studies, and reflect on the lessons learned to enhance your proficiency in this essential econometric technique.
Conclusion
In conclusion, mastering multivariate regression analysis equips students with a powerful tool to navigate the complexities of econometrics homework and contribute meaningfully to empirical research. By understanding the foundational principles, assumptions, and techniques discussed in this handbook, students can confidently tackle real-world problems, analyze data with rigor, and derive valuable insights to inform decision-making in diverse fields. Through diligent practice, engagement with case studies, and continuous learning, students can further refine their skills and become adept econometricians capable of addressing complex economic questions and driving innovation in research and policy. With perseverance and dedication, the journey of mastering multivariate regression analysis becomes a rewarding endeavor with boundless opportunities for growth and impact.
Similar Blogs
Discover insightful articles on economics at EconomicsHomeworkHelper.com. Our blog explores essential concepts, practical applications, and current trends in economics. Whether you're a student aiming to deepen your understanding or a professional seeking valuable insights, our curated content provides expert guidance. Stay informed and excel in economics with our comprehensive blog.