- Understanding Panel Data
- Variables in Panel Data
- Models for Panel Data Analysis
- Pooled OLS (Ordinary Least Squares)
- Fixed Effects Models
- Random Effects Models
- First-Difference Models
- Dealing with Panel Data Issues
- Endogeneity
- Heteroscedasticity
- Serial Correlation
- Missing Data
- Applications of Panel Data Analysis
- Economic Studies
- Healthcare Research
- Educational Research
- Marketing and Business Studies
- Tips for Student Assignments
- Selecting the Appropriate Model
- Addressing Endogeneity
- Checking Assumptions
- Conclusion
Panel data, a dynamic statistical method, has become increasingly influential across diverse academic domains such as economics, sociology, and epidemiology. Navigating the complexities of panel data analysis poses a formidable challenge for university students, yet mastering this technique promises rich rewards. This comprehensive blog aims to demystify panel data analysis, guiding students through its fundamental concepts, applications, and crucial insights to empower them in effectively addressing assignments in this sophisticated statistical realm. Panel data, also known as longitudinal or cross-sectional time-series data, presents observations on multiple entities over numerous time periods, offering a unique perspective that combines individual and temporal effects. Two main types of panel data—balanced and unbalanced—present distinct challenges and opportunities for analysis. Understanding variables within panel data, including dependent, independent, time, and cross-sectional variables, lays the groundwork for robust statistical exploration. In terms of modeling, options such as Pooled OLS, Fixed Effects Models, Random Effects Models, and First-Difference Models each offer distinct approaches to handling individual and time-specific effects. Recognizing the prevalence of issues like endogeneity, heteroscedasticity, serial correlation, and missing data is essential for students embarking on panel data assignments. Techniques like instrumental variable methods, robust standard errors, and Maximum Likelihood Estimation serve as powerful tools in mitigating these challenges. The applications of panel data analysis are vast and impactful, spanning economic studies exploring policy impacts, healthcare research investigating treatment effectiveness, educational studies assessing long-term outcomes, and marketing research examining consumer behavior. Whether you need help with your Econometrics homework or are delving into the intricacies of panel data analysis, this guide aims to equip you with the knowledge and skills to navigate this complex statistical terrain with confidence.
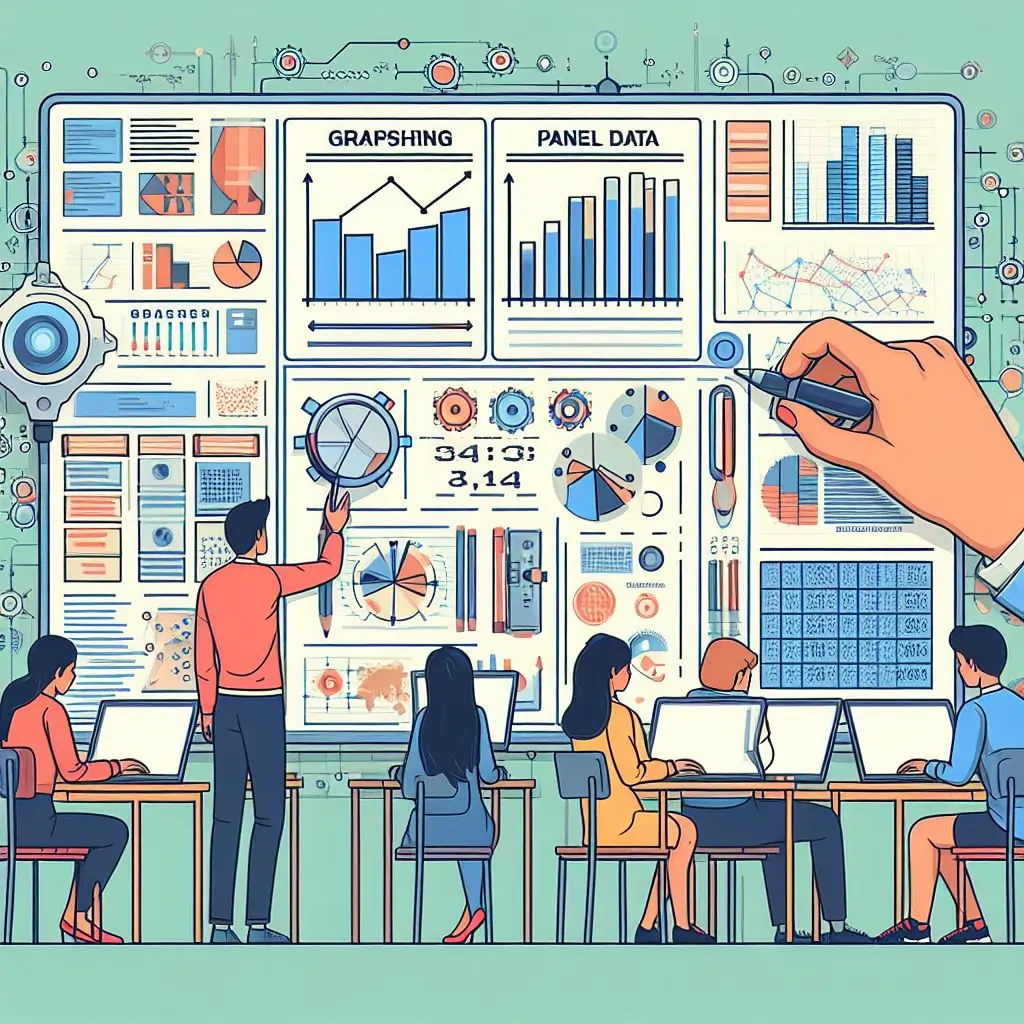
As students engage with panel data assignments, selecting the appropriate model, addressing endogeneity concerns, checking assumptions, and interpreting results are paramount. Choosing the right model, considering the nature of data and associated assumptions, ensures the reliability and validity of results. Rigorous attention to endogeneity issues and the implementation of instrumental variable techniques contribute to the robustness of findings. Regular checks for assumptions, such as homoscedasticity and the absence of serial correlation, safeguard the integrity of the analysis. Ultimately, interpreting results with an eye towards practical implications and acknowledging limitations completes the cycle of effective panel data analysis. In conclusion, this blog equips students with the knowledge and insights necessary for navigating the challenges of panel data analysis, offering a roadmap to success in university assignments and contributing to a deeper understanding of dynamic phenomena across various academic disciplines.
Understanding Panel Data
Understanding panel data is essential for delving into its nuanced statistical analyses. Panel data, synonymous with longitudinal or cross-sectional time-series data, entails observations across multiple subjects or entities spanning various time periods. What sets panel data apart from its counterparts is its capability to capture both individual and time effects, providing a more comprehensive analytical framework. The two primary types of panel data—balanced and unbalanced—play a pivotal role in shaping the analytical landscape. In balanced panels, every subject is observed in each time period, fostering consistency and allowing for direct comparisons. On the other hand, unbalanced panels accommodate variations in the number of observations across time, acknowledging the dynamic nature of real-world data. This foundational understanding of panel data lays the groundwork for students to explore its applications, models, and potential challenges, empowering them in their academic journey.
Variables in Panel Data
In the realm of panel data, understanding key variables is fundamental to conducting meaningful analyses. The dependent variable stands as the focal point of interest, representing what the researcher aims to explain or predict. Complementing this, independent variables play a pivotal role as factors influencing the dependent variable. Notably, these can be categorized as time-invariant (fixed) or time-varying (random), providing a nuanced understanding of the dynamic relationships within the dataset. The time variable is crucial in panel data analysis, representing the specific time periods during which observations are made. This temporal dimension allows researchers to discern trends and patterns over time, offering valuable insights into the evolution of phenomena. Simultaneously, the cross-sectional variable serves to represent individual entities or subjects within the dataset. This facet facilitates the examination of variations across different entities, contributing to a comprehensive understanding of the dataset's intricacies. In essence, these variables collectively form the foundation for rigorous panel data analysis, guiding researchers in uncovering the complexities inherent in their datasets.
Models for Panel Data Analysis
Embarking on panel data analysis involves navigating a diverse landscape of models, each designed to capture distinct aspects of the data's complexity. Pooled OLS (Ordinary Least Squares) serves as a fundamental approach, treating panel data as a cross-sectional dataset and overlooking individual and time effects. While straightforward, this method may introduce bias if unobserved factors influence both the dependent variable and independent variables. Fixed effects models provide a remedy, incorporating fixed dummy variables for individual entities to control for unobservable characteristics that persist over time. However, these models fall short in capturing time-varying individual effects. On the other hand, random effects models assume that individual-specific effects are random and uncorrelated with the independent variables, offering increased efficiency but relying on stringent assumptions. First-difference models present an alternative by focusing on changes over consecutive time periods for each entity, effectively eliminating individual-specific effects. This approach proves valuable when tackling issues related to unobserved heterogeneity. As students delve into panel data assignments, selecting the appropriate model becomes a critical decision. The nature of the data, the presence of individual or time effects, and the assumptions associated with each model demand careful consideration. In this realm, the choice of model can significantly impact the validity and reliability of the results, emphasizing the importance of a thorough understanding of these models for successful panel data analysis.
Pooled OLS (Ordinary Least Squares)
Pooled OLS, a foundational method in panel data analysis, simplifies the complexity of longitudinal data by treating it akin to a standard cross-sectional dataset. This approach, while straightforward, tends to overlook individual and time effects, potentially resulting in biased estimates. By assuming that unobserved individual or time-specific factors are absent, Pooled OLS may provide an oversimplified representation of the dynamics within the dataset. Researchers must exercise caution when using this method, understanding its limitations in capturing nuanced variations over time and across entities.
Fixed Effects Models
In contrast to Pooled OLS, fixed effects models delve deeper into panel data intricacies by incorporating fixed dummy variables for each entity. This model accounts for individual-specific effects, acknowledging that certain characteristics remain constant over time. While effective in controlling for unobservable factors specific to each entity, fixed effects models have limitations, particularly in their inability to capture time-varying individual effects. Consequently, when temporal variations are substantial, researchers might need to explore alternative modeling approaches to ensure a more comprehensive analysis.
Random Effects Models
Random effects models introduce a level of sophistication by assuming that individual-specific effects are random and unrelated to the independent variables. This model offers increased efficiency compared to fixed effects models, as it considers variability across entities. However, this efficiency comes at the cost of assuming that individual effects are not correlated with the independent variables. Researchers must carefully evaluate the appropriateness of this assumption based on the specific characteristics of their dataset.
First-Difference Models
First-difference models offer a distinctive solution by focusing on changes between consecutive time periods for each entity. This approach effectively eliminates individual-specific effects, making it particularly useful when confronting challenges related to unobserved heterogeneity. By differencing the data, researchers create a transformed dataset where the emphasis is on variations over time rather than individual-specific characteristics. This technique is valuable when researchers seek to isolate temporal changes and mitigate the impact of constant individual-specific effects.
Dealing with Panel Data Issues
Navigating the intricate terrain of panel data analysis requires a vigilant approach to addressing common issues that may arise in the dataset. A. Endogeneity, a prevalent concern, surfaces when independent variables correlate with the error term. To counter this, researchers often turn to instrumental variable techniques, ensuring the reliability of their results by identifying variables that are exogenous to the system. Simultaneously, employing fixed effects models can offer a safeguard against endogeneity, capturing unobservable factors that remain constant over time. B. Heteroscedasticity, the uneven variance of the error term across observations, poses a threat to the efficiency of estimates. Researchers combat this by resorting to robust standard errors or more advanced techniques like generalized least squares (GLS), which adaptively adjust for varying error variances. C. Serial Correlation, the presence of correlated error terms across time periods, demands attention. Techniques such as incorporating lagged dependent variables or deploying autoregressive models prove effective in mitigating serial correlation challenges. D. Missing Data, an omnipresent issue, necessitates strategic handling to prevent biased results. Imputation methods or more sophisticated approaches like Maximum Likelihood Estimation (MLE) emerge as powerful tools in addressing the gaps in observational data. As students engage with panel data assignments, a comprehensive understanding of these issues becomes paramount. Rigorous attention to endogeneity, heteroscedasticity, serial correlation, and missing data ensures the robustness and validity of their analyses, paving the way for nuanced interpretations and impactful contributions to the broader field of study. By mastering the techniques to address these challenges, students not only enhance the quality of their assignments but also equip themselves with valuable skills for future endeavors in empirical research and data analysis.
Endogeneity
Endogeneity poses a significant challenge in panel data analysis, emerging when independent variables are correlated with the error term. This correlation can lead to biased estimates and undermine the validity of the results. To tackle endogeneity, researchers often employ instrumental variable techniques or resort to fixed effects models. Instrumental variables serve as proxies that are unrelated to the error term but correlated with the independent variable of interest, providing a robust strategy to disentangle the causal relationship.
Heteroscedasticity
Heteroscedasticity, characterized by unequal variance of the error term across observations, introduces inefficiencies and can distort the precision of parameter estimates. Researchers address this issue by utilizing robust standard errors or employing advanced techniques such as generalized least squares (GLS). Robust standard errors adjust for heteroscedasticity, providing more accurate standard errors in the presence of uneven variances.
Serial Correlation
Serial correlation, the correlation of error terms across different time periods, challenges the independence assumption in panel data. To address this issue, researchers often incorporate lagged dependent variables or employ autoregressive models. Lagged dependent variables introduce the temporal dimension, allowing the model to account for the correlation structure inherent in the data.
Missing Data
Handling missing data is a pervasive concern in panel data analysis. Techniques like imputation, which involves estimating missing values based on observed data, offer a practical solution. Alternatively, employing advanced methods like Maximum Likelihood Estimation (MLE) allows researchers to estimate parameters directly from the likelihood function, accommodating missing observations and enhancing the robustness of the analysis. Adequate handling of missing data ensures the integrity of results and contributes to the reliability of conclusions drawn from panel data studies.
Applications of Panel Data Analysis
The versatility of panel data analysis extends across various fields, enriching research in economics, healthcare, education, and business. A. Economic Studies leverage panel data to dissect the impact of policy changes, economic shocks, and external factors on key indicators such as GDP growth, inflation rates, and unemployment. The ability to track changes over time and across entities provides a nuanced understanding of the complex dynamics within economic systems. B. Healthcare Research benefits from panel data analysis to assess the long-term effectiveness of medical treatments, monitor the progression of diseases, and evaluate the impact of healthcare policies over time. Researchers can discern patterns and trends, informing evidence-based healthcare decisions. C. Educational Research exploits panel data to delve into the enduring effects of educational interventions, investigate the influence of socio-economic factors on academic performance, and trace trends in student outcomes across multiple time periods. Panel data facilitates a comprehensive examination of the evolving educational landscape. D. Marketing and Business Studies employ panel data analysis to scrutinize consumer behavior, identify market trends, and evaluate the efficacy of marketing strategies over time. This dynamic approach allows businesses to adapt their tactics in response to changing market dynamics. As students engage with panel data assignments, recognizing these diverse applications underscores the methodology's real-world impact. Whether unraveling economic intricacies, enhancing healthcare strategies, refining educational interventions, or optimizing business approaches, panel data analysis proves to be an indispensable tool in extracting meaningful insights from complex datasets. The interdisciplinary nature of its applications not only emphasizes its importance in academic research but also highlights its practical relevance in informing decision-making across diverse sectors.
Economic Studies
In the realm of economic studies, panel data analysis stands as a cornerstone, offering a robust framework to dissect the intricate dynamics of economic systems. This methodology is extensively employed to scrutinize the impact of policy changes, economic shocks, and various factors on key economic indicators such as GDP growth, inflation rates, and unemployment. By embracing panel data, economists gain the ability to track changes over time and across entities, fostering a nuanced understanding of the multifaceted forces shaping economic landscapes. The longitudinal and cross-sectional aspects of panel data prove invaluable in unveiling patterns that contribute to informed economic decision-making.
Healthcare Research
Within healthcare research, panel data analysis emerges as a powerful tool for assessing the effectiveness of medical treatments, monitoring the progression of diseases, and evaluating the impact of healthcare policies over time. The ability to analyze data longitudinally facilitates a comprehensive examination of health-related outcomes, enabling researchers to discern trends and patterns that inform evidence-based healthcare strategies. Panel data proves essential in navigating the complexities of healthcare systems and addressing questions related to patient outcomes and policy efficacy.
Educational Research
Educational researchers leverage panel data analysis to gain insights into the long-term effects of educational interventions, understand the impact of socio-economic factors on academic performance, and track trends in student outcomes over time. This methodology allows for a detailed exploration of the evolving educational landscape, offering a dynamic perspective on the factors influencing student success and educational outcomes. By harnessing panel data, researchers contribute to the development of targeted interventions and policies aimed at improving educational quality and equity.
Marketing and Business Studies
In the realm of marketing and business studies, panel data analysis serves as a cornerstone for understanding consumer behavior, identifying market trends, and evaluating the effectiveness of marketing strategies over multiple time periods. Businesses harness the power of panel data to uncover patterns in consumer preferences, track shifts in market dynamics, and assess the long-term impact of marketing initiatives. This approach provides a strategic advantage by enabling companies to adapt their marketing strategies in response to evolving market conditions, ultimately enhancing their competitive edge. The dynamic nature of panel data analysis proves indispensable for businesses seeking to make data-driven decisions and stay ahead in the ever-changing landscape of the marketplace.
Tips for Student Assignments
Embarking on panel data assignments necessitates a strategic approach to ensure thorough and accurate analyses. A. Selecting the Appropriate Model emerges as a critical first step. Careful consideration of the nature of the data, the presence of individual or time effects, and the assumptions associated with each model is imperative. The choice between Pooled OLS, Fixed Effects Models, Random Effects Models, or First-Difference Models significantly influences the reliability and validity of the results. B. Addressing Endogeneity requires meticulous attention. Researchers must recognize the potential correlation between independent variables and the error term, employing instrumental variable techniques or fixed effects models to mitigate bias and enhance the robustness of estimates. C. Checking Assumptions serves as an ongoing task throughout the analysis. Regular scrutiny for issues such as heteroscedasticity, serial correlation, and the normality of residuals ensures the integrity of the results. Robustness checks, including sensitivity analyses, further validate the findings and contribute to the overall rigor of the assignment. D. Interpreting Results demands a nuanced understanding of the implications. Students should delve beyond statistical significance and assess the practical importance of their findings. Acknowledging the limitations of the chosen model and discussing potential implications for real-world scenarios adds depth to the interpretation. In conclusion, navigating panel data assignments requires a combination of theoretical knowledge, methodological acumen, and critical thinking skills. By selecting the appropriate model, addressing endogeneity concerns, checking assumptions rigorously, and interpreting results judiciously, students can elevate their analyses and contribute meaningfully to the understanding of dynamic phenomena in their respective fields of study. As the field of panel data analysis continues to evolve, these tips serve as valuable guides, empowering students to navigate the challenges and complexities inherent in this sophisticated statistical methodology.
Selecting the Appropriate Model
The foundation of a successful panel data analysis lies in selecting the right model. Careful consideration of the nature of the data is paramount. Assess whether the data is balanced or unbalanced, and evaluate the presence of individual or time effects. Each model, be it Pooled OLS, Fixed Effects Models, Random Effects Models, or First-Difference Models, comes with distinct assumptions and applications. Understanding these nuances is crucial in choosing a model that aligns with the specific characteristics of the dataset. Rigorous model selection ensures that the analysis captures the complexities of the underlying phenomena, leading to more accurate and meaningful results.
Addressing Endogeneity
Endogeneity poses a persistent challenge in panel data analysis, potentially compromising the validity of results. Being mindful of this issue is crucial. Researchers should explore instrumental variable techniques as a remedy. Instrumental variables, when carefully selected, can serve as proxies that are unrelated to the error term but correlated with the independent variable of interest, helping disentangle causality and mitigate bias. This step is essential to fortify the reliability of the results, especially in scenarios where endogeneity may lurk beneath the surface.
Checking Assumptions
Regularly scrutinizing assumptions is a fundamental aspect of panel data analysis. Issues such as homoscedasticity, normality of residuals, and the absence of serial correlation demand vigilant attention. Robustness checks, including sensitivity analyses, contribute to the validation of findings. Ensuring that these assumptions hold true enhances the robustness and credibility of the analysis. Researchers should be diligent in this regard, as overlooking assumption violations can undermine the integrity of the results.
Conclusion
Interpreting the results of panel data analysis requires more than a statistical understanding; it demands a grasp of the practical implications. Researchers should take the time to delve into the meaning behind the numbers. Consider the broader context and implications of the findings, connecting them to real-world scenarios. Additionally, acknowledging and discussing any limitations is crucial for a comprehensive interpretation. This step adds depth to the analysis, providing a nuanced understanding that extends beyond statistical significance, ultimately contributing to a more insightful and meaningful exploration of the data.
Similar Blogs
Get expert help with your economics homework at economicshomeworkhelper.com. Our blog offers valuable insights, tips, and resources to enhance your understanding and performance in economics. Stay updated with the latest trends and improve your academic success with our expert guidance.